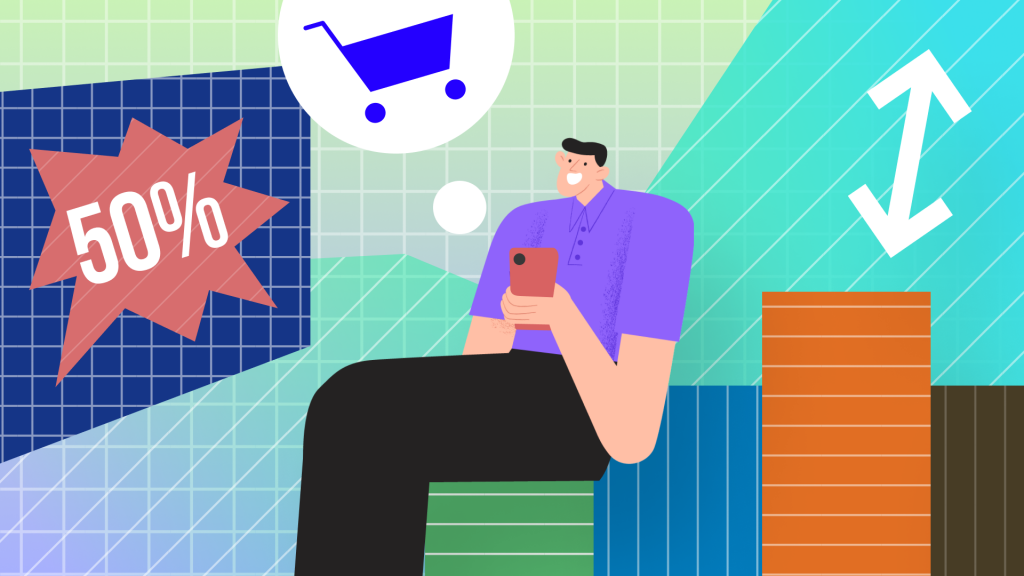
Marketing mix modeling is a well-established and widely used standard approach for measuring key drivers of sales or any other business metric. In our previous article we provided a detailed explanation of how this tool works and compared our solution with Robyn, the open-source tool by Meta. Here you will learn about how MMM is used to measure the long-term effects of advertising.
MMM allows estimating the incremental increase in sales, driven by both media features (through the nonlinear transformation of their metrics — response curves) and other external factors (macroeconomic indicators, pricing, promotions, distribution, etc.). They are particularly useful and are usually applied for identifying the short and medium-term impact of marketing
This approach works well for products in mature stages or those that have reached a certain level of brand-awareness. However, products in the growth or market entry stages often require a different approach. Brand-building through creating an emotional connection between the consumer and the brand forms enduring consumer preferences and directly influences the upward trend in sales.
To understand and evaluate the real effectiveness of marketing in such cases, it is necessary to assess not only short and medium-term but also long-term marketing effects.
Approaches to measuring the long-term marketing effects
The marketing literature has extensively explored the connection between advertising and consumer demand, considering both short-term and long-term effects (Binet & Field,2019, Neslinetal.,1994; Wittink et al.,1988).
Deterministic trend
The simplest abstraction of long-term marketing effects is the growth of base sales, driven by the increase in brand recognition metrics. In the traditional classical marketing mix models, base sales are considered a flat value, and the easiest approach to account for the growth of base sales is to use a deterministic trend.
This trend can be evaluated using time series decomposition techniques such as Seasonal Decomposition of Time Series on Loess (Cleveland et al., 1990) or Decomposable Time Series Models (Harvey & Peters 1990). However, a deterministic trend provides only a fixed and deterministic view, revealing nothing about the long-term demand influenced by marketing as there are no direct connections between them in the model.
Two-step modeling framework
A much more sophisticated approach involves combining consumer mindset metrics (such as unaided awareness and consideration) with the path-to-purchase process using a two-step modeling framework. This framework combines Unobserved Components techniques for short-term effects estimation with cointegrated VAR analysis for the long-term impact of marketing (Cain P. 2022).
While this decision is more complex, it provides a specific theoretical foundation for long-term brand building and allows capturing the complete sales conversion process. However, it requires consumer mindset metrics observations, which may only be available on a monthly basis or may not be available at all.
AdoptoMedia approach
To address this problem, we propose a straightforward and simpler extension of our existing modeling framework, where consumer mindset data is not required. We assume that each subsequent campaign in a specific media channel may contribute to sales increment cumulatively.
The nonlinearity of this effect is accounted for by the same response curve filter function used for short-term effects estimation. We also assume that minimum lag effects are restricted to be no less than the estimated lag for the short-term impact of a specific media channel.
The final response curve equation is as follows:
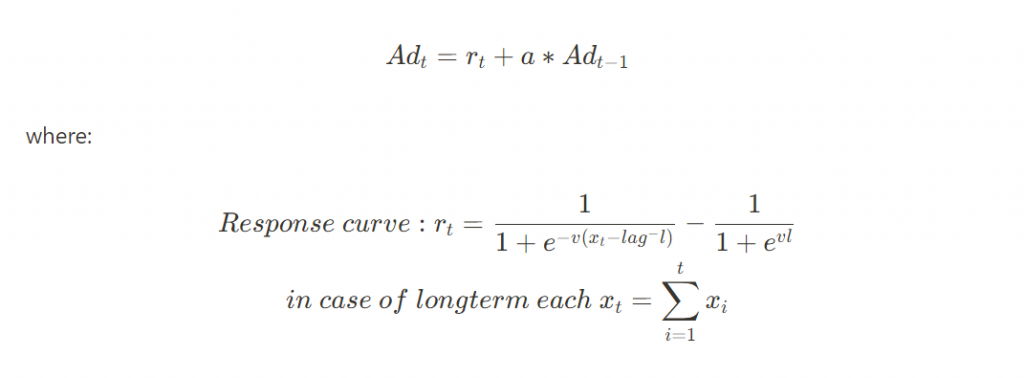
“a” is an adstock parameter, “v” and “l” are curve shape and saturation threshold parameters, respectively.
Parameter choice is automated by combining multi-objective hyperparameter optimization using a Hyperband Hyperparameter Optimization algorithm, a probabilistic-based feature selection technique, and several model validation procedures to provide a set of optimal results with well-tuned response curve hyperparameters in a fairly short turnaround time.
A multicollinearity problem, where multiple media channels are currently on air delivered at the same time or during the same periods, is addressed by using additional scale hyperparameters to normalized response curve time series along with the ability to account for a priori estimates of the impact/effectiveness of the media channels received as a result of other trustworthy research.
Calculating the long-term effects: case study
Let’s see how this can look in practice: the following e-commerce product case uses 2 media channels according to their current marketing strategy: TV and Digital
We also can observe a clear trend in the data after the campaign launch:
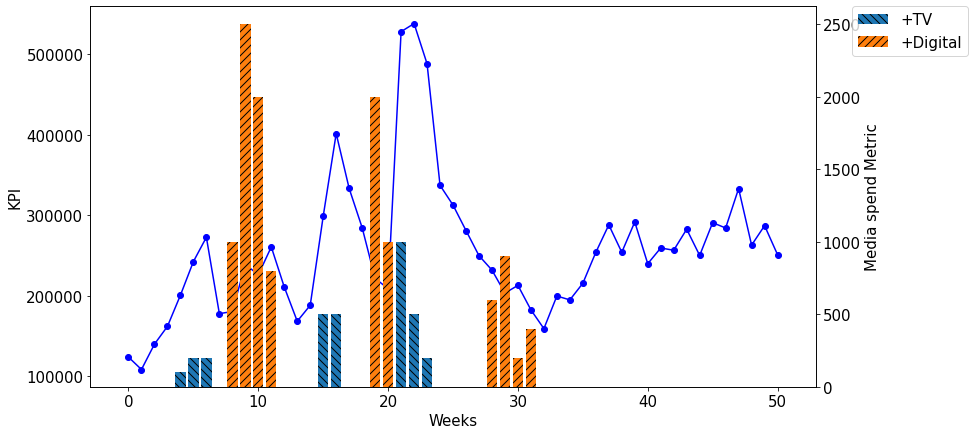
We’ve implemented an additive marketing mix model to estimate both short and long-term effects of media, taking into account additional promotional data (discount depth) and seasonality index which is based on internet queries over the last two years. The seasonal component is estimated using a supplementary decomposable time series model and transformed through min-max normalization. Weekly data is provided for only 52 weeks, with the first 47 observations used for training, and the last 5 for evaluating the predictive ability of the model. Statistical test results are presented at the end of the article in Fig.5
Upon training the model, we can evaluate the complete incremental effect of each channel both in the short term and as a long-term base growth.
The decomposition of the model and its fit quality are illustrated in the figures below:
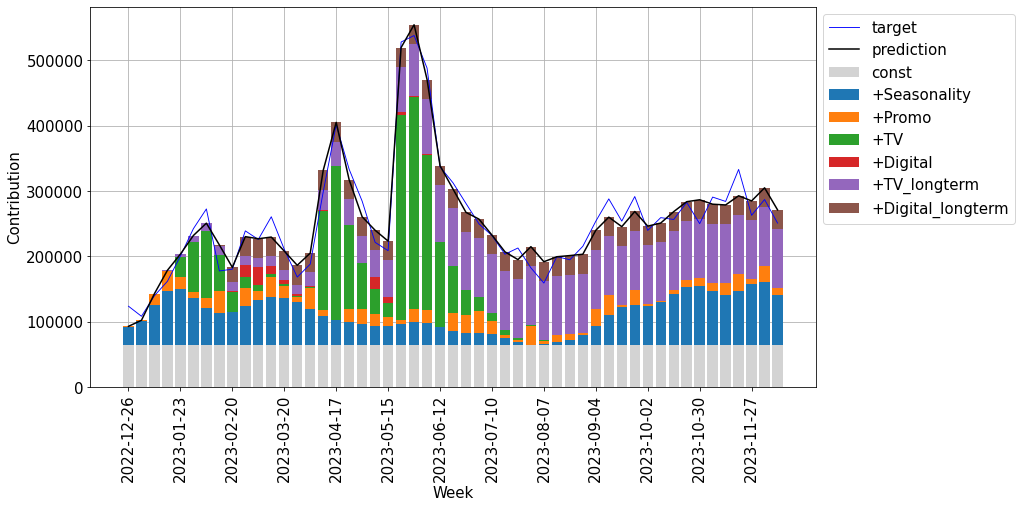
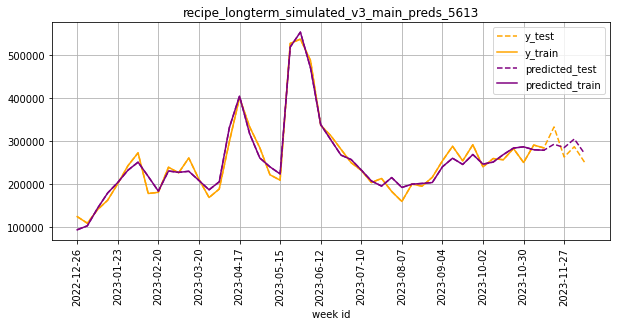
The model allows us to access media channel response curves to get further insights about the saturation level of each individual channel:
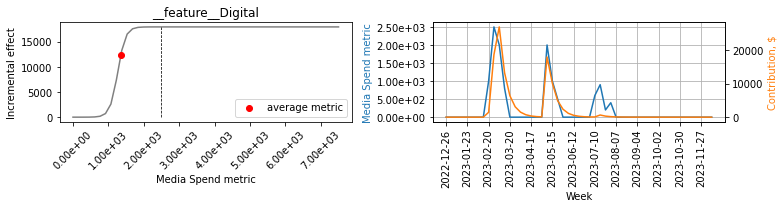
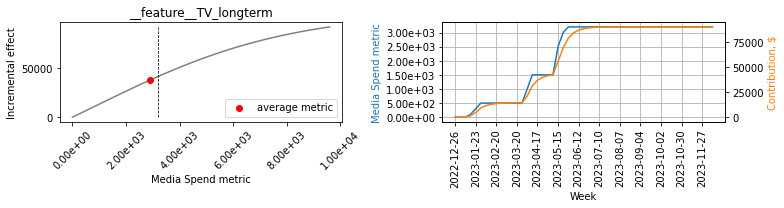
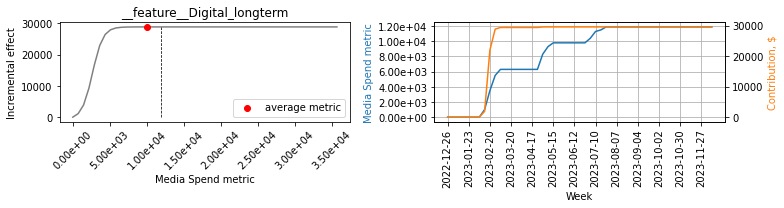
Summary statistical test results:
‘MAPE_train’: 6.2%
‘MAPE_test’: 8.7%
‘acorr_ljungbox min lb_pvalue’: 0.42
‘Shapiro-Wilk test p-value’: 0.21
‘Reset Ramsey test Quadratic only p-value’: 0.89
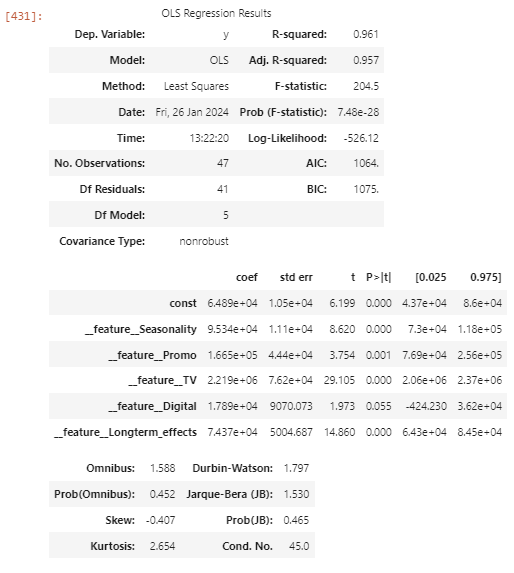
Conclusion
Measuring the long-term effect of advertising is important to get the full picture of the company’s marketing performance.
Using all information obtained from MMM we can combine the individual impact of each media channel to calculate the total marketing contribution to sales broken down into short and long-term uplift and ROAS thereby giving a significant advantage over classic marketing mix models with deterministic trends.
When evaluating the long-term effects of advertising, it is also important to consider that the trend could be caused by other drivers such as macroeconomic indicators. That is why during discussions with the business it is crucial to identify and formulate hypotheses about possible growth drivers before defining the model specification and its training.